Quick Start
RLLTE provides reliable implementations for highly-recognized RL algorithms, and users can build applications with very simple code.
On NVIDIA GPU
Suppose we want to use DrQ-v2 to solve a task of DeepMind Control Suite, and
it suffices to write a train.py
like:
train.py
# import `env` and `agent` module
from rllte.env import make_dmc_env
from rllte.agent import DrQv2
if __name__ == "__main__":
device = "cuda:0"
# create env, and `eval_env` is optional
env = make_dmc_env(env_id="cartpole_balance", device=device)
eval_env = make_dmc_env(env_id="cartpole_balance", device=device)
# create agent
agent = DrQv2(env=env,
eval_env=eval_env,
device='cuda',
tag="drqv2_dmc_pixel")
# start training
agent.train(num_train_steps=5000, log_interval=1000)
Run train.py
and you will see the following output:
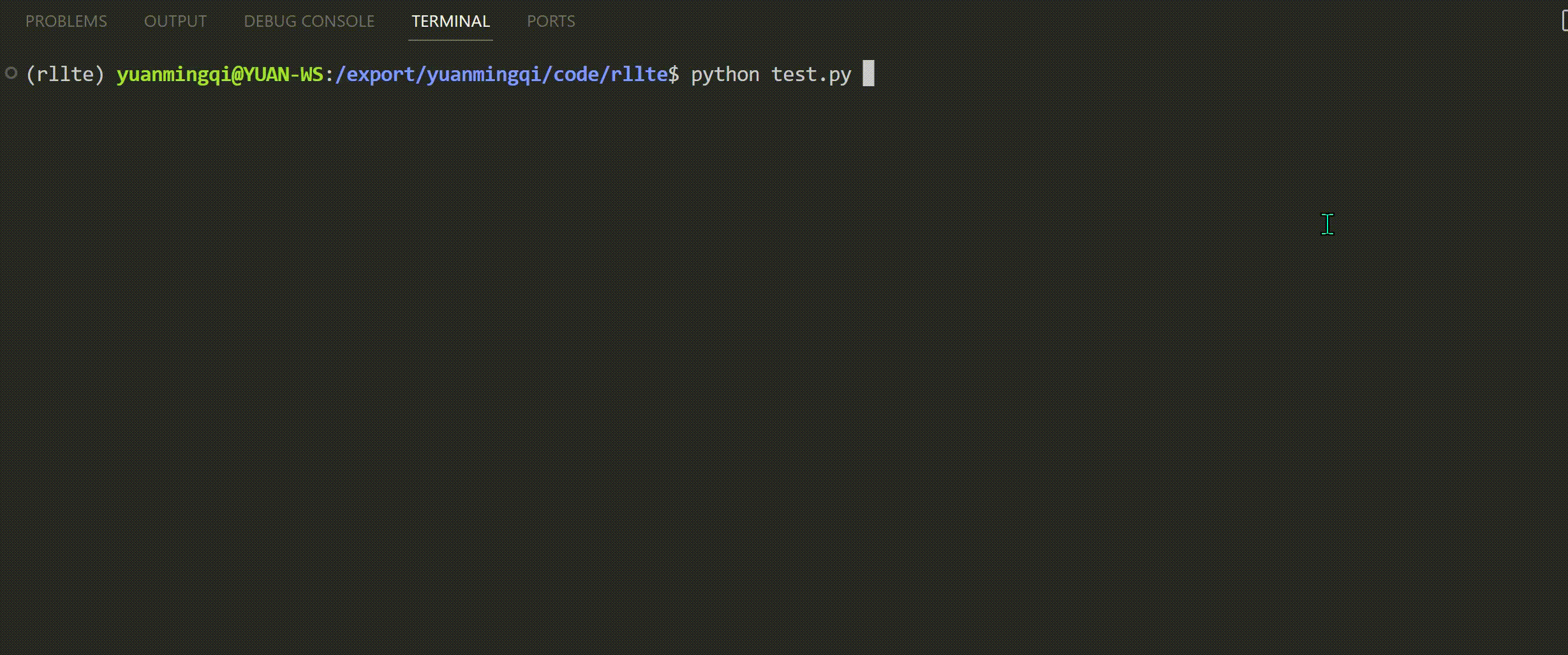
Read the logs
- S: Number of environment steps. Note that
S
isn't equal to the number of frames in visual tasks, andnumber_of_frames=number_of_steps * number_of_action_repeats
- E: Number of environment episodes.
- L: Average episode length.
- R: Average episode reward.
- FPS: Training FPS.
- T: Time costs.
On HUAWEI NPU
Similarly, if we want to train an agent on HUAWEI NPU, it suffices to replace cuda
with npu
:
Compatibility of NPU
Please refer to https://docs.rllte.dev/api/ for the compatibility of NPU.
Load the trained model
Once the training is finished, you can find agent.pth
in the subfolder model
of the specified working directory.